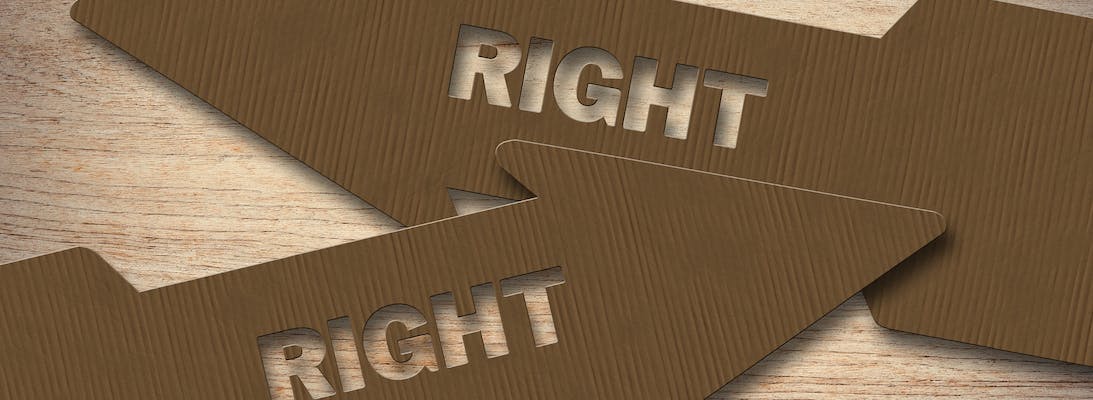
Data Factory - Dynamic mappings in Data Flow
Azure Data Factory and Data Flows make transforming data from one format to another super simple with it's code free approach. However that doesn't mean we want to construct entire data flows when changing mappings from one value to another.
For example if my source data contained a field for favourite creature with value An for Ants, and Ca for Cats I could do the transformation using an iif expression in a derived column task. But in a few weeks time if I needed to add Ba for Bats, editing the whole Data Flow seems like a lot of work, not to mention nested iif statements are going to be come ugly and confusing.
One option would be to have the list of conversions as another source in the flow and do a join, but then this means having that data stored somewhere like blob storage.
Instead a solution I have is to pass the data in as a parameter to the data flow. Data Factory doesn't have an array parameter but we can put a comma separated list in as a string. e.g. An=Ants, Ba=Bats, Ca=Cats.
Then in our derived columns expression we can do this:
1iif(instr($ParameterString, toString(SourceValue) + "=")==0,"No Mapping",2 substring(3 substring($ParameterString, instr($ParameterString, toString(SourceValue) + "=")),4 length(toString(SourceValue))+2,5 iif(instr(substring($ParameterString,instr($ParameterString, toString(SourceValue) + "=")+length(toString(SourceValue))+2),",") > 0,6 instr(substring($ParameterString,instr($ParameterString, toString(SourceValue) + "=")+length(toString(SourceValue))+2),","),7 length(substring($ParameterString,instr($ParameterString, toString(SourceValue) + "=")))8 )9 )10 )
There's quite a lot going on here so lets break it down
1iif(instr($ParameterString, toString(SourceValue) + "=")==0,"No Mapping",
First we are doing an iif to check if our Source Value (the value from our dataset) exists within the Parameter String. If it doesn't then we're setting the value to "No Mapping"
1 substring(
If the value does exist then we need to grab just the part we want. So we need a sub string and we need to get everything after the source values equals to the next comma.
A reminder the parameters for substring are substring(<string to subset>: string,<from 1-based index>: integral, [<number of characters>: integral])
1 substring($ParameterString, instr($ParameterString, toString(SourceValue) + "=")),
Our first parameter needs to be the string to subset, that's going to come from our ParameterString, but we're going to do another substring on it to ignore everything before the one we ant to match.
So if our ParameterString was set too:
An=Ants, Ba=Bats, Ca=Cats
and our Source Value was Ba we would now have:
Ba=Bats, Ca=Cats
1 length(toString(SourceValue))+2,
Next is the start index which will be the length of our source value + 2. If we ended the substring now we would get.
Bats, Ca=Cats
1 iif(instr(substring($ParameterString,instr($ParameterString, toString(SourceValue) + "=")+length(toString(SourceValue))+2),",") > 0,2 instr(substring($ParameterString,instr($ParameterString, toString(SourceValue) + "=")+length(toString(SourceValue))+2),","),3 length(substring($ParameterString,instr($ParameterString, toString(SourceValue) + "=")))4 )5 )
The final parameter for the substring is a bit more complex, but it's similar to what we have just done.
Two scenarios need to be catered for:
- If there's more items in the list left then there will be a comma separating them. If this is the case then we need to get the position of the first comma in what we have left.
- If there isn't anything else in the list then there will be no comma. In this instance we need to get the length of what remains.
With that our substring will now return Bats